Modeling Feedback and Gaze in Human-Robot Interaction
Compared to a written chat, spoken face-to-face interaction is a much richer form of communication, relying not only on words but also on a variety of non-verbal cues. Elements like prosody, facial expressions, and gaze play crucial roles in giving feedback, aligning understanding, regulating turn-taking, and conveying emotions. This project focuses on modeling these non-verbal aspects of human communication. This research is motivated not only by a desire to deepen our understanding of human communication but also by the goal of enhancing human-robot interactions, with the aim of creating robots that are more socially aware and responsive.
Gaze in conversation
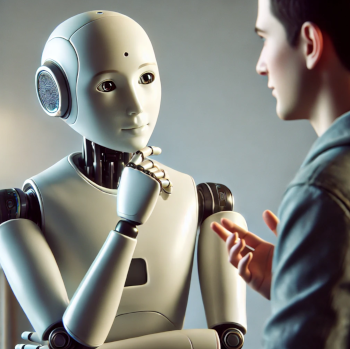
Gaze behavior is a fundamental component of human interaction, serving multiple communicative functions, such as signaling attention, managing turn-taking, and indicating the focus of interest. In human-robot interactions, accurately modeling gaze patterns can help robots better interpret and predict conversational cues. In our project, we explore how we can best model the gaze behavior of robots, and how the robot’s gaze influences the interaction.
Feedback and Backchannels
Feedback in conversations is often non-verbal, for example in the form of head nods or vocal backchannels - brief vocalizations (e.g., "mm-hmm," "uh-huh") that indicate understanding, agreement, or interest. These signals are crucial for smooth and natural dialogue flow, as they provide reassurance to the speaker and help align conversational goals. In human-robot interaction, it is crucial to both understand feedback from the user to the robot, in order for the robot to be able to adapt to the user, as well as being able to accurately produce appropriate feedback, reflecting the robot’s level of understanding.
Here is an example of how we are using unsupervised learning to allow machines to automatically "discover" various feedback functions:
You have previously denied the display of content of the type "External media". Do you want to show content?
Adaptive robot presenters
To explore how a robot could make use of feedback from the user, we have created a test-bed where a robot is presenting a piece of art to a human audience. The robot then detects feedback from the audience in order to adapt the presentation according the human's level of understanding.
This is explained in the following video (automatically generated from the slides):
You have previously denied the display of content of the type "External media". Do you want to show content?
Publications
Funding
- Representation Learning for Conversational AI (WASP, 2021-2026)
- COBRA: Conversational Brains (EU MSCA ITN, 2020-2023)
- COIN: Co-adaptive human-robot interactive systems (SSF, 2016-2020)
Researchers
