Topics:
• Distributed file systems
• No SQL databases
• Scalable messaging systems
• Big Data execution engines: Map-Reduce, Spark
• High level queries and interactive processing: Hive and Spark SQL
• Stream processing
• Graph processing
• Scalable machine learning
• Resource management
FID3019 Advanced course in Data-Intensive Computing 7.5 credits
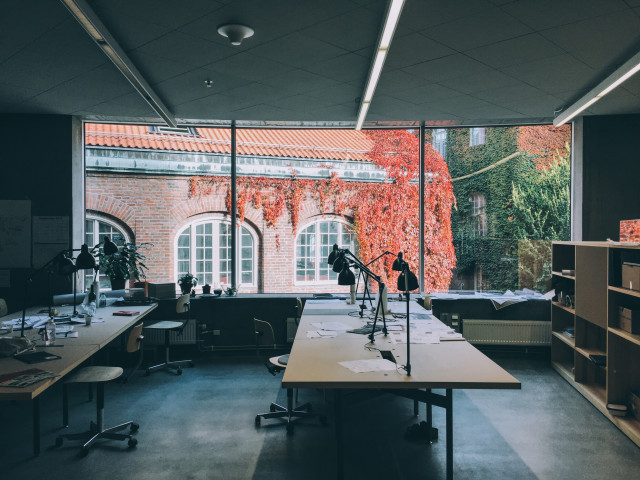
Information per course offering
Information for Autumn 2024 Start 26 Aug 2024 programme students
- Course location
KTH Kista
- Duration
- 26 Aug 2024 - 27 Oct 2024
- Periods
- P1 (7.5 hp)
- Pace of study
50%
- Application code
51307
- Form of study
Normal Daytime
- Language of instruction
English
- Course memo
- Course memo is not published
- Number of places
Places are not limited
- Target group
- No information inserted
- Planned modular schedule
- [object Object]
- Schedule
- Part of programme
- No information inserted
Contact
Course syllabus as PDF
Please note: all information from the Course syllabus is available on this page in an accessible format.
Course syllabus FID3019 (Spring 2019–)Information for research students about course offerings
On a yearly basis during the fall semester (period 1)
Content and learning outcomes
Course contents
Intended learning outcomes
The course complements distributed systems courses, with a focus on processing, storing and analyzing massive data. It prepares the students for Ph.D. studies in the area of data-intensive computing systems.
The main objective of this course is to provide the students with a solid foundation for understanding large scale distributed systems used for storing and processing massive data.
More specifically after the course is completed the student will be able to:
• Explain the architecture and properties of the computer systems needed to store, search and index large volumes of data.
• Describe the different computational models for processing large data sets for data at rest (batch processing) and data in motion (stream processing).
• Use various computational engines to design and implements nontrivial analytics on massive data.
• Explain the different models for scheduling and resource allocation computational tasks on large computing clusters.
• Elaborate on the tradeoffs when designing efficient algorithms for processing massive data in a distributed computing setting.
Literature and preparations
Specific prerequisites
Enrolled as a doctoral student.
Recommended prerequisites
Recommended prerequisites: Basic knowledge in distributed systems and programming models, programming languages (Scala, Java, Python)
Equipment
Literature
Examination and completion
If the course is discontinued, students may request to be examined during the following two academic years.
Grading scale
Examination
- EXA1 - Examination, 7.5 credits, grading scale: P, F
Based on recommendation from KTH’s coordinator for disabilities, the examiner will decide how to adapt an examination for students with documented disability.
The examiner may apply another examination format when re-examining individual students.
P/F
Other requirements for final grade
The course will be assessed with a Pass/Fail grade, based on a successful presentation, the delivery of a scientifically sound report, and the identification of appropriate papers for the reading list. In addition to this, students must attend at least 75% of all seminars.
Opportunity to complete the requirements via supplementary examination
Opportunity to raise an approved grade via renewed examination
Examiner
Ethical approach
- All members of a group are responsible for the group's work.
- In any assessment, every student shall honestly disclose any help received and sources used.
- In an oral assessment, every student shall be able to present and answer questions about the entire assignment and solution.