- Deep networks.
- Probabilistic deep learning.
- Deep transfer and sharing of knowledge.
- Unsupervised deep representation learning.
- Higher order learning.
- Adversarial learning.
FDD3412 Deep Learning, Advanced Course 6.0 credits
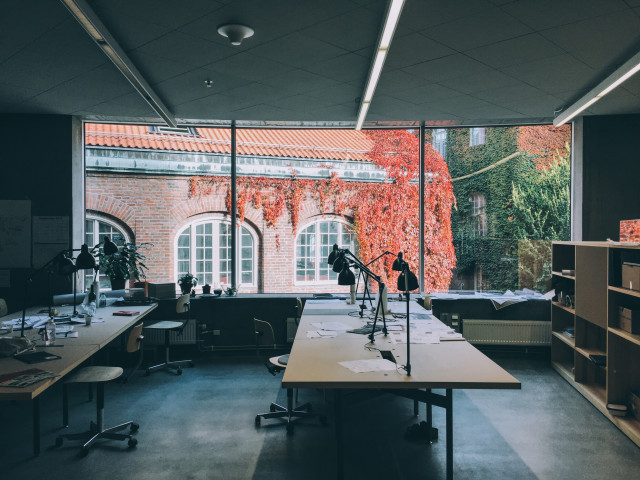
This course is going to be replaced by another course from HT25 with a course ID of FDD3610. For more information refer to the master level course DD2610 which will be run in conjunction.
The course goes beyond the basic principles of deep learning by delving into the frontiers of deep learning research.
Information per course offering
Course offerings are missing for current or upcoming semesters.
Course syllabus as PDF
Please note: all information from the Course syllabus is available on this page in an accessible format.
Course syllabus FDD3412 (Spring 2019–)Content and learning outcomes
Course contents
Intended learning outcomes
After passing the course, the students should be able to:
- explain and justify the subareas of deep learning,
- account for the theoretical background for advanced deep learning techniques,
- identify the directions in which additional research can be made to develop the field,
- implement methods based on recently published results,
- analyse advanced research in the area and critically evaluate the methods' weaknesses and strengths
in order to:
- prepare for degree project/postgraduate studies in deep learning,
- become better trained to meet industry's need of key competence in the area.
Literature and preparations
Specific prerequisites
Recommended prerequisites
Completed course DD2424 Deep Learning in Data Science or DD2437 Artificial Neural Networks and Deep Architectures or the equivalent courses.
Literature
Uppgift om kurslitteratur meddelas i kurs-PM.
Examination and completion
Grading scale
Examination
- EXA1 - Exam, 6.0 credits, grading scale: P, F
Based on recommendation from KTH’s coordinator for disabilities, the examiner will decide how to adapt an examination for students with documented disability.
The examiner may apply another examination format when re-examining individual students.
If the course is discontinued, students may request to be examined during the following two academic years.
Other requirements for final grade
Passing grade on the laboratory work and passing grade on the final project.
Examiner
Ethical approach
- All members of a group are responsible for the group's work.
- In any assessment, every student shall honestly disclose any help received and sources used.
- In an oral assessment, every student shall be able to present and answer questions about the entire assignment and solution.