The course focuses on specialized hardware implementation architectures of deep learning algorithms for resource-constrained dependable embedded systems. It also discusses system architectures exploiting deep leaming.
Course memo Autumn 2021
Course presentation
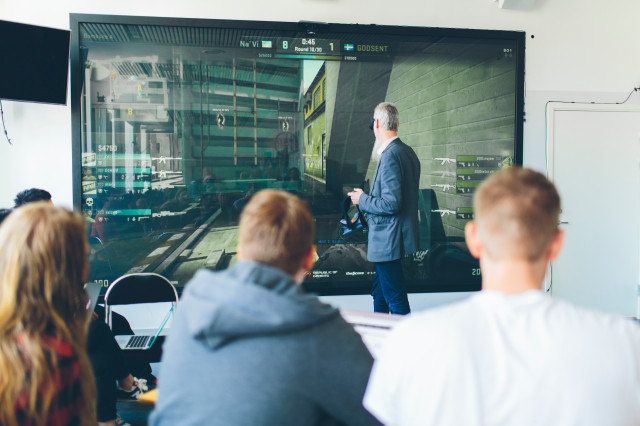
Headings denoted with an asterisk ( * ) is retrieved from the course syllabus version Autumn 2021
Content and learning outcomes
Course contents
The course consists of two modules. Module I introduces basic knowledge in machine learning and algorithms for deep learning Module II focuses on specialised hardware implementation architectures for deep learning algorithms and new brain-like computer system architectures. Apart from presenting relevant informative knowledge, the course contains laboratory and project assignments to create understanding of the related algorithms applied to deal with real problems and to contrast and evaluate alternative implementation architectures, in term of performance, cost, and reliability.
Module I: Algorithms for deep learning
Module I introduces basic machine learning algorithms, basic neural network algorithms and algorithms for deep learning. Among a number of machine learning algorithms, this module introduces the algorithms for linear regression, polynomial regression, logistic regression that are fundamental and most relevant for neural networks. For neural networks we consider perceptrons, multi-layer-perceptrons and in particular the back-propagation algorithm. After presenting traditional statistical learning machine learning and neural networks this module further examplifies deep learning algorithms, specifically Convolutional Neural Networks (CNN) and Recurrent Neural Networks (RNN).
Module II: Architecture specialization for deep learning
Module II examines specialised hardware based implementation architectures for deep learning algorithms. From a broad spectrum of potential hardware architectures the design alternatives, such as GPGPU:s, domain specific processors, FPGA/ASIC-based accelerators are presented, together with their advantages and disadvantages. In particular limitations and design alternatives for using deep learning algorithms in embedded resource constrained systems will be discussed. Furthermore this module will discuss new architectures in deep learning for computer system design such as brain-like computer system architectures. A case study with analysis, evaluation and application of a deep learing architectures will be carried out.
Intended learning outcomes
After passing the course, the student should be able to
- describe and explain basic neural networks and deep learning algorithms and their relations
- explain and justify the hardware design space for deep learning algorithms
- choose and apply an appropriate deep learning algorithm, to solve real problems with artificial intelligence in embedded systems
- analyse and evaluate hardware implementation alternatives for deep learning algorithms
- suggest and justify an implementation architecture for applications with deep learning in embedded resource constrained systems
- discuss and comment new hardware implementation architectures for deep learning and new brain-like computer system architectures that utilise new devices and new concepts
in order to
- understand the necessity, importance, and potential of accelerating deep learning algorithms with low power consumption through specialized hardware architecture
- discuss, suggest and evaluate specialised hardware architectures to implement deep learning algorithms and utilise deep learning concepts in resource constrained reliable systems.
Learning activities
The course consists of 11 lectures, 3 labs, 2 seminars and 1 exercise (student recitation). The labs and seminars are group works. The exercise is individual work.
Detailed plan
Learning activities | Content | Preparations |
---|---|---|
Lecture 1. Course introduction and motivation | This lecture introduces the course objectives, course content and structure, and course assessment. We also motivate hardware acceleration for deep learning. |
Visit and review all content in the Canvas course room. Read slides for Lecture 1 on the Canvas course room. |
Lecture 2. Linear regression and logistic regression | This lecture introduces two basic statistical learning models starting from linear regression to logistic regression. | Pre-review slides for Lecture 2 on the Canvas course room. |
Lecture 3. Perceptron and Multi-Layer Perceptron (MLP) | This lecture discusses the general concepts of artificial neural networks (ANNs) from perceptron, general neuron model to multi-layer perceptron (MLP), in particular, about network training and inference. | Pre-review slides for Lecture 3 on the Canvas course room. |
Lecture 4. Lecture 4 CNN (Convolutional Neural Network) | This lecture presents Convolutional Neural Network as one very successful example of Deep Neural Networks (DNNs). | Pre-review slides for Lecture 4 on the Canvas course room. |
Lab 1. Hardware Design, Implementation and Evaluation of Artificial Neuron |
In this lab, the tasks are to design three RTL models (three alternative ways) for implementing an N-input artificial neuron. After you verify their correct functionality, you bring the designs for logic synthesis. |
Try to finish the lab tasks. |
Lecture 5. RNN (Recurrent Neural Network) | This lecture presents another important category of DNN, namely Recurrent Neural Network (RNN) which considers neuron interactions over time with memory effect. | Pre-review slides for Lecture 5 on the Canvas course room. |
Lecture 6. Hardware acceleration for deep learning: Challenges and Overview; Model minimization I | This lecture discusses the efficiency challenges (performance, power/energy, resource) of executing deep learning algorithms on hardware, and opens the problem space for hardware acceleration of deep learning algorithms. We discuss the model minimization issues such as network reduction, data quantization, compression, fixed-point operations etc. for efficient hardware implementations of neural network algorithms. | Pre-review slides for Lecture 6 on the Canvas course room. |
Lecture 7. Model minimization II | This lecture continues discussing latest model minimization techniques: Network pruning, Data quantization and approximation, and Network sparsity. | Pre-review slides for Lecture 7 on the Canvas course room. |
Lecture 8. Hardware Specialization I | This lecture discusses hardware specializations for neural network algorithms, focusing on digital hardware design and compute system architecture design principles. | Pre-review slides for Lecture 8 on the Canvas course room. |
Lab 2. Convolutional Neural Networks for Image Classification in PyTorch | This lab prepares you with necessary skills and knowledge for performing basic deep learning tasks in PyTorch. In particular, you are going to realize CNNs for image classification. | Try to finish the lab tasks. |
Seminar I. Deep Learning and Minimization of Neural Network Models | A workshop in a conference setting. Each student group is both a presenter (presenting its assigned paper) and an opponent (asking questions to another group). | Read the assigned paper, prepare presentation slides as a group, and prepare questions to another group. |
Lecture 9. Hardware specialization II | This lecture continues to discuss in-depth latest techniques used for hardware acceleration: sparsity computing and ASIP. | Pre-review slides for Lecture 9 on the Canvas course room. |
Lecture 10. Model-to-Architecture Mapping and EDA | This lecture discusses model to architecture mapping, its optimization and Electronic Design Automation (EDA). | Pre-review slides for Lecture 10 on the Canvas course room. |
Lecture 11. Technology-Driven Deep Learning Acceleration and Brain-Like Computing or Invited Lecture | This lecture gives an outlook of efficient hardware acceleration of neural networks, in particular, with a focus on technology impact such as embedded DRAM, 3D stacking, memresistor etc, and also brain-inspired computer. This lecture may be organized as an invited lecture to cover latest research and development in the field. | Pre-review slides for Lecture 11 on the Canvas course room. |
Exercise (Student recitation) | This is a student recitation session. The exercise questions are collected in an exercise compendium. The questions cover all lectures. | Finish the exercise questions individually before the exercise session. |
Lab 3 | For this lab, you can choose one of the two tasks: (1) Hardware design, implemenation and evaluation of MLP; (2) Transfer Learning and Network Pruning. | Try to finish the lab tasks. |
Seminar II. Case studies of deep learning hardware accelerators | A workshop in a conference setting. Each student group is both a presenter (presenting its assigned paper) and an opponent (asking questions to another group). | Read the assigned paper, prepare presentation slides as a group, and prepare questions to another group. |
Preparations before course start
Specific preparations
- Get you familar with the programming language Python (online Python tutorial https://docs.python.org/3/tutorial/ ), and the deep learning framework PyTorch (https://pytorch.org)
- Install Python and Pytorch. For installation, suggestion is to use the package manager Anaconda, which installs all dependencies. https://www.anaconda.com/distribution/
- A good preparation is to follow the tutorial “Deep Learning with PyTorch: A 60 Minute Blitz” to run and understand all the given code.
Literature
No information insertedSoftware
- Hardware (ASIC/FPGA) synthesis tool with license.
- Programming language: Python.
- Deep Learning framework: Pytorch.
Support for students with disabilities
Students at KTH with a permanent disability can get support during studies from Funka:
Examination and completion
Grading scale
A, B, C, D, E, FX, F
Examination
- LAB1 - Laboratory work, 3.0 credits, Grading scale: P, F
- TEN1 - Written exam, 4.5 credits, Grading scale: A, B, C, D, E, FX, F
Based on recommendation from KTH’s coordinator for disabilities, the examiner will decide how to adapt an examination for students with documented disability.
The examiner may apply another examination format when re-examining individual students.
The section below is not retrieved from the course syllabus:
Laboratory work ( LAB1 )
Written exam ( TEN1 )
- You need to complete both LAB1 and TEN1 in order to complete the course.
- Upon the completion of both LAB1 and TEN1, the grade of the written examination will be the course grade.
Ethical approach
- All members of a group are responsible for the group's work.
- In any assessment, every student shall honestly disclose any help received and sources used.
- In an oral assessment, every student shall be able to present and answer questions about the entire assignment and solution.
Further information
Changes of the course before this course offering
Fine adjustment on the detaied plan is possible, but the detailed plan will be settled before the course offering.
Contacts
Course Coordinator
Teachers
Teacher Assistants
Examiner
Round Facts
Start date
1 Nov 2021
Course offering
- TEBSM Autumn 2021-51310
Language Of Instruction
English