New algorithm makes machine learning faster and more accurate
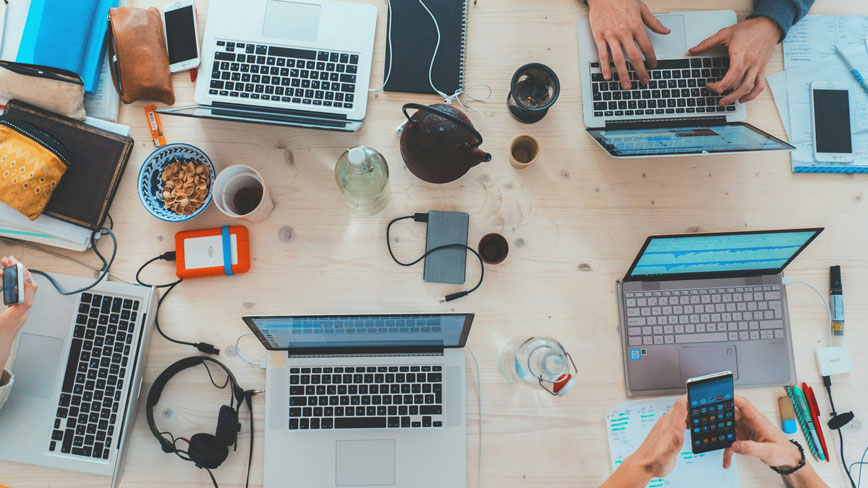
The algorithm can improve the way smart devices in homes and workplaces work together. By reducing the frequency with which devices need to talk to a server while seamlessly handling different data between devices.
In a paper published at the 2024 International Conference on Acoustics, Speech, and Signal Processing , KTH researchers Jiaojiao Zhang and Mikael Johansson, together with Jiang Hu from Harvard University, present a cutting-edge algorithm designed to tackle the current challenges of Federated Learning (FL).
Traditional methods are limited
Federated Learning has emerged as an important framework in machine learning, empowering collaborative model training across multiple organizations and users without any need to share the underlying data.
However, traditional FL methods are limited in what problems they can solve, and have encountered hurdles in managing communication bottlenecks and dealing with data diversity among clients.
Enter the innovative algorithm proposed by Zhang, Hu and Johansson.
By separating data handling and communication, it makes FL more efficient. It not only allows to solve regularized FL problems but it also reduces the frequency of communication between devices and the server, rendering the whole process faster.
Moreover, it can handle diverse data among clients without any problems.
Three key features
“This work represents a significant leap forward in Federated Learning, which in turn can enhance smart device capabilities in homes and workplaces by enabling more efficient collaborative model training using distributed data.”
Key features of the algorithm include:
• it is able to handle regularized FL problems efficiently, both with respect to computations and communications.
• it ensures high accuracy of trained models even when dealing with diverse data among devices.
• it reduces communication frequency between devices and the server, speeding up the whole learning process.
Zhang, Hu and Johansson received the ICASSP2024 Best Paper Award for their work, “showcasing innovation and academic excellence in acoustics, speech, and signal processing.”
Read the article Composite Federated Learning with Heterogeneous Data
Text: Sturle Hauge Simonsen ( sturle@kth.se )
Related news
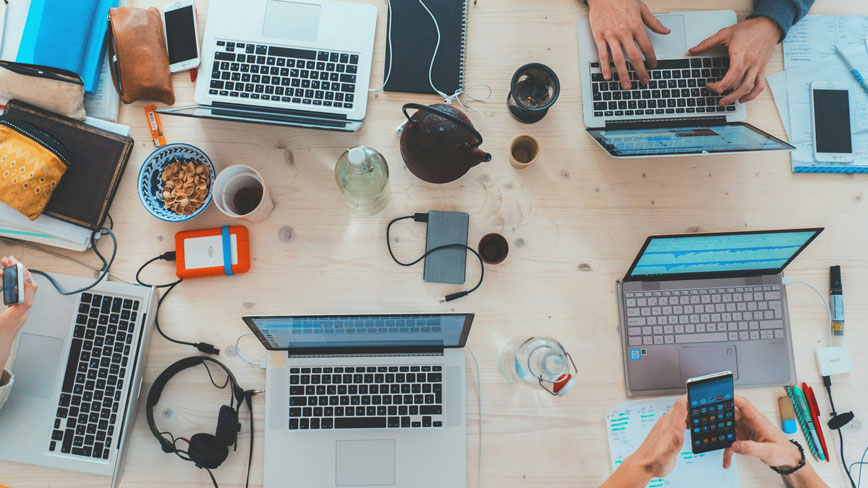
New algorithm makes machine learning faster and more accurate
The algorithm can improve the way smart devices in homes and workplaces work together. By reducing the frequency with which devices need to talk to a server while seamlessly handling different data be...
Read the article
Studying traffic flows in Kista to reduce emissions
To better understand emissions from traffic, researchers will use big data and AI to study traffic flows in Kista. The hope is to create transport solutions that generate lower emissions and reduce th...
Read the article