Improved wireless communication can boost launch of autonomous vehicles

Collaboration with Princeton University merges machine learning with cellular networks, making cars safer and more energy efficient. Together with experts in machine learning and wireless communication, KTH postdoc José Mairton Barros Da Silva Júnior work on a project that will accelerate the development of safe and reliable autonomous vehicles.
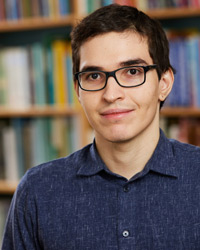
With funding from the EU’s New Horizon 2020 programme, FLASH (Federated Learning Supporting Efficient and Reliable Inference over Vehicular Networks) will develop learning algorithms to handle data sets better, improve the capacity to predict changes in their surroundings and adhere to the reliability requirements associated with self-driving cars.
The project is led by professor Carlo Fischione and his group at the Division of Network and Systems Engineering at KTH and professor H. Vincent Poor at the Department of Electrical and Computer Engineering at Princeton University.
Mairton Barros has big dreams for the project. “In the next five or ten years, I hope it has contributed to the realization of autonomous vehicles,” he says.
Cars learning from each other
FLASH will apply a learning technique called federated learning to solve prediction tasks between multiple autonomous vehicles. The method uses distributed data generated by the sensors placed on all the cars. Essentially, they will be trained to learn from each other using the data they generate from their sensors. This will make it easier for self-driving cars to predict the movements of other vehicles and how pedestrians and cyclists move.
“My research focuses on solving the problems that occur when we combine federated learning with cellular communication networks. This is an increasingly exciting field because of rapid improvements in distributed machine learning and the launch of 5G cellular networks.”
The project, he believes, will help make cars more energy efficient, lower emissions, and reduce fuel consumption, contributing to the fulfilment of the UN Sustainable Development Goals. More reliable algorithms will also improve real-time decisions that reduce the risk of traffic accidents and fatalities.
Dr Mairton Barros looks forward to collaborating with colleagues from Princeton University.
“This is a unique opportunity to establish new scientific collaborations and networking on an international scale. Hopefully, that can foster novel research on integrating federated learning and vehicular communications,” he concludes.