- Generative models.
- Bayesian inference.
- Probabilistic programming.
- Graphical models.
- Concealed Markov models with continuous states.
- Particle filters.
- Monte Carlo estimation.
- Sequential Monte Carlo.
- Markov Chain Monte Carlo.
- Clustering.
- The Dirichlet process.
DD2447 Statistical Methods in Applied Computer Science 6.0 credits
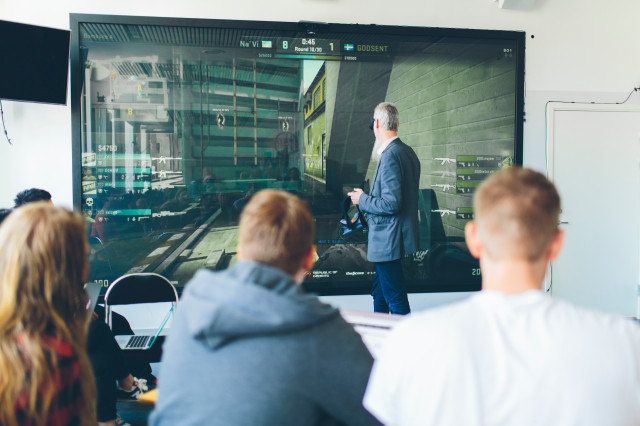
This course summarizes statistical and probabilistic methods used in applied Computer Science.
Information per course offering
Choose semester and course offering to see current information and more about the course, such as course syllabus, study period, and application information.
Information for Autumn 2025 statmet25 programme students
- Course location
KTH Campus
- Duration
- 27 Oct 2025 - 12 Jan 2026
- Periods
- P2 (6.0 hp)
- Pace of study
33%
- Application code
52038
- Form of study
Normal Daytime
- Language of instruction
English
- Course memo
- Course memo is not published
- Number of places
Places are not limited
- Target group
Open for all students from year 3 and for students admitted to a master's programme, as long as it can be included in your programme.
- Planned modular schedule
- [object Object]
- Schedule
- Schedule is not published
- Part of programme
Master's Programme, Computer Science, åk 2, CSCS, Recommended
Master's Programme, Computer Science, åk 2, CSDA, Recommended
Master's Programme, Computer Science, åk 2, CSTC, Recommended
Master's Programme, Cybersecurity, åk 1, Recommended
Master's Programme, Cybersecurity, åk 2, Recommended
Master's Programme, ICT Innovation, åk 1, DASC, Recommended
Master's Programme, ICT Innovation, åk 1, DASE, Recommended
Master's Programme, ICT Innovation, åk 2, DASC, Recommended
Master's Programme, ICT Innovation, åk 2, DASE, Recommended
Master's Programme, Industrial Engineering and Management, åk 1, MAIG, Conditionally Elective
Master's Programme, Machine Learning, åk 1, Conditionally Elective
Master's Programme, Machine Learning, åk 2, Conditionally Elective
Master's Programme, Mathematics, åk 1, Optional
Master's Programme, Systems, Control and Robotics, åk 2, Recommended
Contact
Jens Lagergren, e-post: jensl@kth.se
Course syllabus as PDF
Please note: all information from the Course syllabus is available on this page in an accessible format.
Course syllabus DD2447 (Autumn 2022–)Content and learning outcomes
Course contents
Intended learning outcomes
After passing the course, the student shall be able to
- explain and justify several important methods for machine learning
- give an account of several types of methods and algorithms that are used in the field of sample-based inference methods
- implement several types of methods and algorithms that are used in the field based on a high-level description
- extend and modify the methods that the course deals with
in order to be able to make a degree project in sample-based inference methods.
Literature and preparations
Specific prerequisites
Knowledge in algebra and geometry, 7.5 higher education credits, equivalent to completed course SF1624.
Knowledge in one variable calculus, 7.5 higher education credits, equivalent to completed course SF1625.
Knowledge in probability theory and statistics, 6 higher education credits, equivalent to completed course SF1910-SF1924/SF1935.
Knowledge and skills in programming, 6 higher education credits, equivalent to completed course DD1310/DD1311/DD1312/DD1314/DD1315/DD1316/DD1318/DD1321/DD1331/DD1337/DD100N/ID1018.
Knowledge in algorithms and data structures, at least 6 higher education credits, equivalent to completed course DD1320/DD1321/DD1325/DD1326/DD1327/DD2325/ID1020/ID1021.
Active participation in a course offering where the final examination is not yet reported in LADOK is considered equivalent to completion of the course.
Being registered for a course counts as active participation.
The term 'final examination' encompasses both the regular examination and the first re-examination.
Recommended prerequisites
Courses in mathematics (analysis), programming, computer science and statistics equivalent to obligatory courses on D- or F-programme.
Matlab or similar tool (Octave, R).
Equipment
Literature
Examination and completion
If the course is discontinued, students may request to be examined during the following two academic years.
Grading scale
Examination
- INL1 - Assignment, 6.0 credits, grading scale: A, B, C, D, E, FX, F
Based on recommendation from KTH’s coordinator for disabilities, the examiner will decide how to adapt an examination for students with documented disability.
The examiner may apply another examination format when re-examining individual students.
Other requirements for final grade
Written assignments and a project (INL1; 6 higher education credits).
Opportunity to complete the requirements via supplementary examination
Opportunity to raise an approved grade via renewed examination
Examiner
Ethical approach
- All members of a group are responsible for the group's work.
- In any assessment, every student shall honestly disclose any help received and sources used.
- In an oral assessment, every student shall be able to present and answer questions about the entire assignment and solution.
Further information
Course room in Canvas
Offered by
Main field of study
Education cycle
Add-on studies
Please discuss with the course leader.
Contact
Supplementary information
In this course, the EECS code of honor applies, see:
http://www.kth.se/en/eecs/utbildning/hederskodex
This course overlaps with DD2420 Probabilistic Graphical Model.